Programmingempire
Since making accurate predictions on the basis of historical data helps us in determining the likely outcome, it is very important for making decisions in nearly all kinds of business. There are several Machine Learning techniques that we can use to predict the outcome given a dataset. This article presents a number of Popular Machine Learning Algorithms for Prediction.
What is Predictive Modeling?
Machine Learning is known for Predictive Modeling. In other words, we can predict the value of a dependent variable y by applying a function f on the independent variable x.
Generally, we use predictive modeling or predictive analytics in order to forecast future outcomes. For this purpose, we systematically collect data about an event. This data is also called historical data. After that, we train a statistical model. We use the trained statistical model to predict future results.
Use of Machine Learning in Prediction
In fact, Machine Learning offers several techniques that we can to make predictions on the basis of historical data. Indeed there are both supervised learning techniques such as Linear Regression as well as unsupervised machine learning techniques such as Long Short Term Memory that we can use for predicting the outcome.
List of Popular Machine Learning Algorithms for Prediction
The following list shows some of the widely used Machine Learning algorithms for prediction.
- Linear Regression is the simplest of all Machine Learning algorithms. Basically, it determines the relationship between the two variables where one is the independent variable and the other one is the dependent variable. However, this algorithm is too simple and may not be appropriate for complex problems.
- Another Machine Learning algorithm that we can use for predictions is the Decision Tree. Basically, the Decision Tree algorithm uses the historic data to build the tree. In order to predict the outcome, the prediction process starts with the root node and examines the branches according to the values of attributes in the data. Accordingly, the process is repeated until a leaf node is reached.
- Although Time Searies Analysis is a statistical technique rather than a machine learning technique, it is also an important tool for making predictive analysis. Basically, it employs techniques such as autocorrelation and moving averages to predict the future values of the dependent variable.
- The K-Means Clustering is an unsupervised Machine Learning technique that takes input dataset without labels. Further, it creates the clusters of data points. After that, we can use these clusters for the classification task. Since, the data points of new records will fall in one of the clusters, it helps us in predicting the outcome.
Use of Neural Networks in Prediction
- In fact, Artificial Neural Networks (ANN), including both forward and backpropagation networks can be used for prediction since we can train them with historical data. Once, the neural network is trained, we can use it to predict the future outcome. However, neural networks are less preferred for predictive analysis since their outcome is harder to predict. Also, they are more complex than the simpler Regression Analysis. Besides, ANNs require a large amount of data for training.
- Unlike ANN, Recurrent Neural Network (RNN) has self-loops in the hidden layers so that it can learn from the previous states of the hidden layers. Therefore, it offers a better understanding of the context. However, RNN suffers from not remembering the long-term dependencies. Once trained with sufficient data, RNN can predict future outcomes.
- Basically, a Long Short Term Memory (LSTM) network is a kind of Recurrent Neural Networks (RNN) that solve the long-term dependency problem by having a Cell State. Accordingly, each cell retains only that information of the past that helps it in learning and discards the rest. For the purpose of making predictions, we can train the network using entires training data and save the model. Later we can load the model and use it to make predictions about the new data.
Further Reading
How to Implement Inheritance in Python
Find Prime Numbers in Given Range in Python
Running Instructions in an Interactive Interpreter in Python
Deep Learning Practice Exercise
Deep Learning Methods for Object Detection
Image Contrast Enhancement using Histogram Equalization
Transfer Learning and its Applications
Examples of OpenCV Library in Python
Understanding Blockchain Concepts
Example of Multi-layer Perceptron Classifier in Python
Measuring Performance of Classification using Confusion Matrix
Artificial Neural Network (ANN) Model using Scikit-Learn
Popular Machine Learning Algorithms for Prediction
Long Short Term Memory – An Artificial Recurrent Neural Network Architecture
Python Project Ideas for Undergraduate Students
Creating Basic Charts using Plotly
Visualizing Regression Models with lmplot() and residplot() in Seaborn
Data Visualization with Pandas
A Brief Introduction of Pandas Library in Python
A Brief Tutorial on NumPy in Python
- Angular
- ASP.NET
- C
- C#
- C++
- CSS
- Dot Net Framework
- HTML
- IoT
- Java
- JavaScript
- Kotlin
- PHP
- Power Bi
- Python
- Scratch 3.0
- TypeScript
- VB.NET
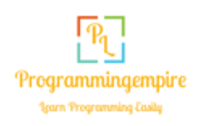